On This Page:
Internal validity refers to whether the design and conduct of a study are able to support that a causal relationship exists between the independent and dependent variables.
It ensures that no other variables except the independent variable caused the observed effect on the dependent variable.
Conducting research that has strong internal and external validity requires thoughtful planning and design from the outset.
Rather than hastening through the design process, it’s wise to invest sufficient time in structuring a study that is methodologically robust and widely applicable.
By carefully considering factors that can compromise internal and external validity during the design phase, one can avoid having to remedy issues later.
Research that exhibits both high internal and external validity permits drawing forceful conclusions about the findings. Though it may require more initial effort, ensuring studies have sound internal and external validity is necessary for producing meaningful and influential research.
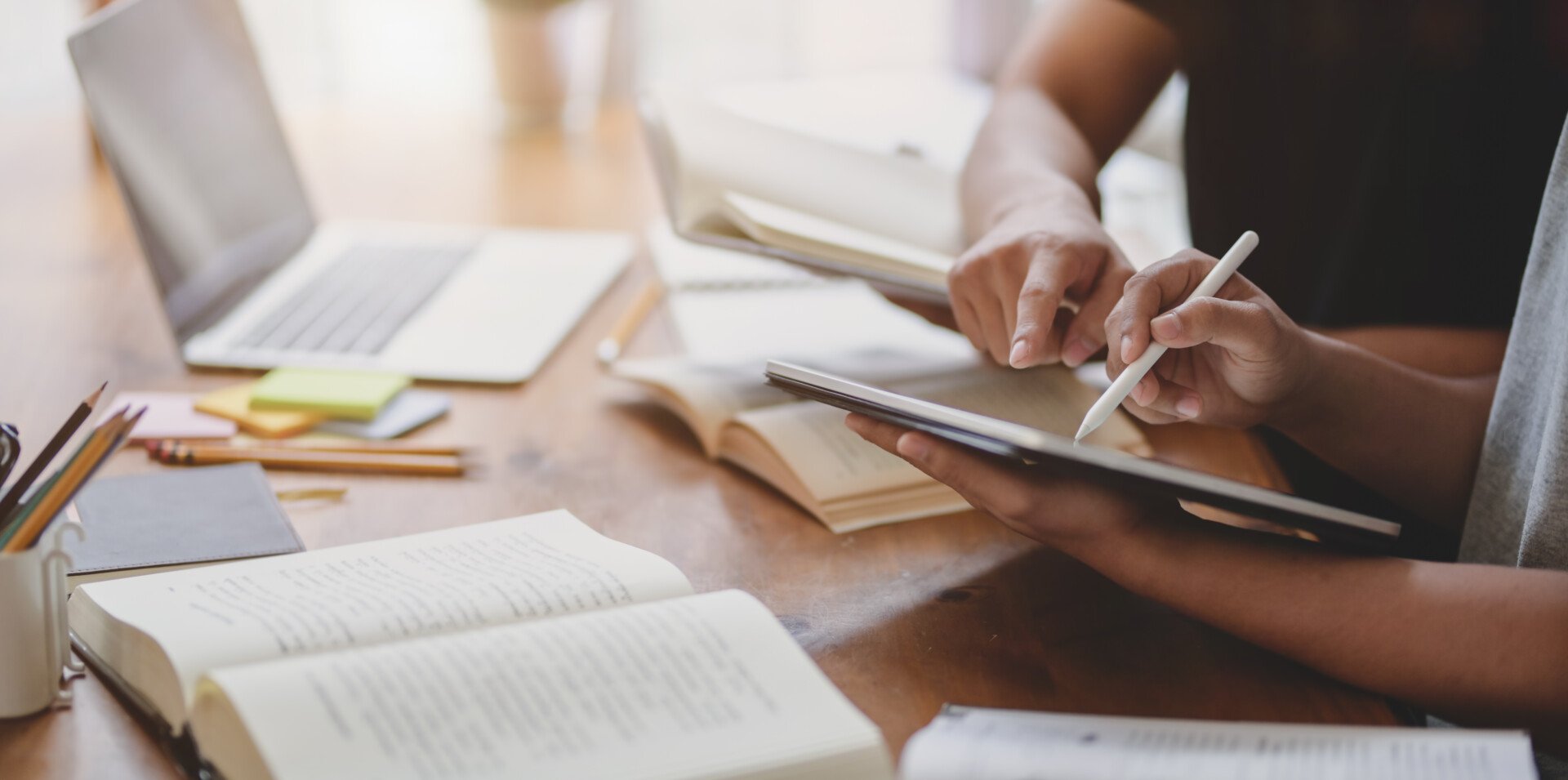
For example, if you implement a smoking cessation program and see improvement among participants, high internal validity means you can be confident this is due to the program itself rather than other influences.
Internal validity is not black-and-white – it’s about the level of confidence we can have in results based on how well the study controls for variables that could undermine the findings.
The more a study avoids potential “confounding factors,” the higher its internal validity and the more faith we can place in the cause-effect relationship it uncovers.
For the general public, internal validity is important because it means a given study’s results and takeaways can be trusted and applied.
Threats to Internal Validity
Confounding variables
Confounding variables are extraneous factors that influence the dependent variables in an experiment, causing a misleading association and making it difficult to isolate the true effect of the independent variable.
They threaten internal validity because they provide alternative explanations for study results, making it unclear if changes in the dependent variable are really due to manipulation of the independent variable or due to the confounding variable.
A failure to control extraneous variables undermines the ability of researchers to create causal inferences logically. Unfortunately, however, confounding variables are difficult to control outside of laboratory settings.
Nonetheless, Campbell (1957) identified several confounding variables that can threaten internal validity.
Participant Factors
Participant reaction biases threaten internal validity because participants may act differently when they know they are being observed. These biases include participant expectancies, participant reactance, and evaluation apprehension.
Participant expectancies occur when a participant, consciously or unconsciously, attempts to behave in a way that the experimenter expects them to. The overly cooperative participant may often base their behavior on factors such as study setting and directions.
Participant expectancies may also occur during a participant screening process. For example, a participant hoping to participate in a study about depression may exaggerate their symptoms on a screening questionnaire to appear more eligible for the study.
Participant reactance occurs when participants intentionally try to act in a way counter to the experimenter’s hypothesis.
For example, if studying the effects of daylight exposure on sleep habits, a participant may intentionally sleep at exactly the same time, regardless of whether or not they are exposed to daylight. Intentional uncooperativeness could result from a desire for autonomy or independence (Brehm, 1966).
Evaluation apprehension happens when a desire to appear consistent with social or group beliefs affects participant responses.
This response style can polarize responses and lead to inappropriate conclusions. For instance, participants asked about their opinions on a political issue in a group may feel pressure to conform to the responses of other group members.
Broadly, researchers can reduce these biases by guaranteeing participant anonymity, using cover stories, unobtrusive observations, and indirect measures.
Sampling bias
Sampling bias occurs when the process of selecting participants for a research study results in key differences between groups that could skew the results. This threatens internal validity because it introduces systematic error in the comparisons between an experimental group and a control group.
For example, let’s say a study is testing a new math tutoring program and students are randomly assigned to either participate in the program (experiment group) or continue with normal instruction (control group).
However, the researcher unknowingly samples students for the experiment group from advanced math classes, while the control group is sampled from regular math classes.
In this case, a sampling bias is introduced because the students in the experiment group may have higher math abilities or motivation levels to begin with compared to the control group.
Any positive effects observed from the tutoring program could simply be due to these pre-existing differences rather than being an actual result of the program itself.
Attrition
According to Campbell (1957), attrition, otherwise known as experimental mortality, refers to a differential loss of study participants in experimental and control groups.
This can threaten internal validity if the rate of attrition differs significantly between the experimental and control groups.
For example, imagine a clinical trial testing the effectiveness of a new therapy for depression. Participants are randomly assigned to either receive the therapy (experimental group) or no therapy (control group) for 8 weeks.
Over the course of the study, a number of participants from both groups drop out and are lost to follow-up. However, twice as many participants dropped out from the control group compared to the experimental group.
This differential attrition introduces bias because the participants remaining in each condition are no longer equivalent – the experimental group now contains more of its original participants compared to the smaller subset remaining in the control group.
Any observed differences in depression levels by the end of the study could be due to this systematic imbalance rather than being an actual effect of the therapy.
Experimenter bias
Experimenter bias refers to when a researcher’s expectations, perceptions, or motivations influence the outcome of an experiment in unconscious ways. This threatens internal validity because it provides an alternative explanation for results besides the independent variable being tested.
For example, a psychologist is conducting an experiment on the effects of praise on child task performance. The psychologist hypothesizes that praising children will improve their task performance.
During the experiment, she unconsciously provided more encouragement and positive body language when interacting with the praise group versus the neutral group.
Consequently, the praise group shows better task performance. However, it is unclear whether this is truly due to the predictive praise or inadvertent experimenter bias, where children picked up on the researcher’s subtle supportive cues.
This demonstrates how a researcher’s cognitive bias can unknowingly impact participant responses and behavior in a way that distorts the causal relationship between variables.
History
History encompasses specific events that a study participant experiences during the course of an experiment that is not part of the experiment itself.
Specifically, it threatens the internal validity of experiments that take place over longer periods of time. For example, imagine a 12-month clinical trial testing a new psychotherapy for reducing anxiety. Participants are randomly assigned to receive either the new therapy or an existing therapy.
However, 8 months into the trial, the COVID-19 pandemic begins. This external event increases anxiety levels for people everywhere.
By the end of the trial, anxiety levels are reassessed. The new therapy group shows greater reductions in anxiety compared to the existing therapy group.
However, it is unclear whether this difference is truly due to the new therapy’s effectiveness or the confounding variable of COVID-19 raising anxiety in the control group.
Perhaps anxiety would have decreased similarly in both groups if not for the pandemic. This demonstrates how history can introduce confounds and alternative explanations that undermine internal validity.
Instrumentation
Instrumentation refers to the ability of experimental instruments to provide consistent results throughout the course of a study.
Instrumentation threats occur when there are changes in the calibration or administration of the tools, surveys, or measures used to collect data over the course of a study.
This can introduce systematic measurement error and provide an alternative explanation for any observed differences aside from the independent variable.
For example, a researcher using a battery-powered device to measure blood pressure in an experiment intended to investigate the effectiveness of a drug in reducing hypertension may find that the battery’s progressive decay may result in these readings appearing lower on a post-test than on the pre-tests.
Instrumentation is not limited to electronic or mechanical instruments. For example, a newly-hired researcher asked to rate the mental health status of participants over the course of a month may, with experience, be able to rate participants more accurately in the post-test than during the pre-test (Flannelly et al., 2018).
Diffusion of information between participants
The diffusion of information and treatments between patients can call internal validity into question. The latter case describes a situation in which research participants adopt a different intervention than the one they were assigned because they believe the different interventions to be more effective.
For example, a control participant in a weight-loss study who learns that those in the treatment group are losing more weight than them may adopt the treatment group’s intervention.
Differential diffusion of information can also occur when participants are given different instructions or instructions that can be misinterpreted by those conducting the study.
For instance, participants asked to take a medication biweekly may take it twice a week or once every two weeks (Flannelly et al., 2018; Campbell, 1957).
Maturation
Maturation encompasses any biological changes related to age, or otherwise that occur with the passage of time. This can include becoming hungry, tired, or fatigued, wound healing, recovering from surgery, and disease progression.
Maturation threatens internal validity because natural changes over time can provide an alternative explanation for study results rather than the independent variable itself.
For example, in a year-long study of a new reading program for children, students may show reading gains over the course of the year. However, some of that improvement could simply be due to neural development and growing reading skills expected with age.
The effects of maturation can also take effect over studies that have a short duration — for example, children given a repetitive computer task may lose focus within an hour, resulting in worsened performance (Flannelly et al., 2018).
Testing
Testing refers to when participants taking a test or assessment can perform better simply from having experienced it before. Familiarity with the test can influence results rather than any intervention or independent variable being studied.
For example, let’s say a researcher is testing a new method for improving memory in older adults. Participants take a memory assessment before and after completing the new memory training program.
However, participants may show memory improvements in the post-test partly just because it was their second time taking the exact same test. Their prior experience with the questions and format benefits their scores.
This demonstrates how repeated testing on the same measures can threaten internal validity. It provides an alternative explanation that improvements were due to practice effects rather than being an actual result of the intervention.
How can we prevent threats to internal validity?
Some methods for increasing the internal validity of an experiment include:
Random allocation
Random allocation is a technique that chooses individuals for treatment groups without regard to researchers’ will or patient condition and preference. This increases internal validity by reducing experimenter and selection bias (Kim & Shin, 2014).
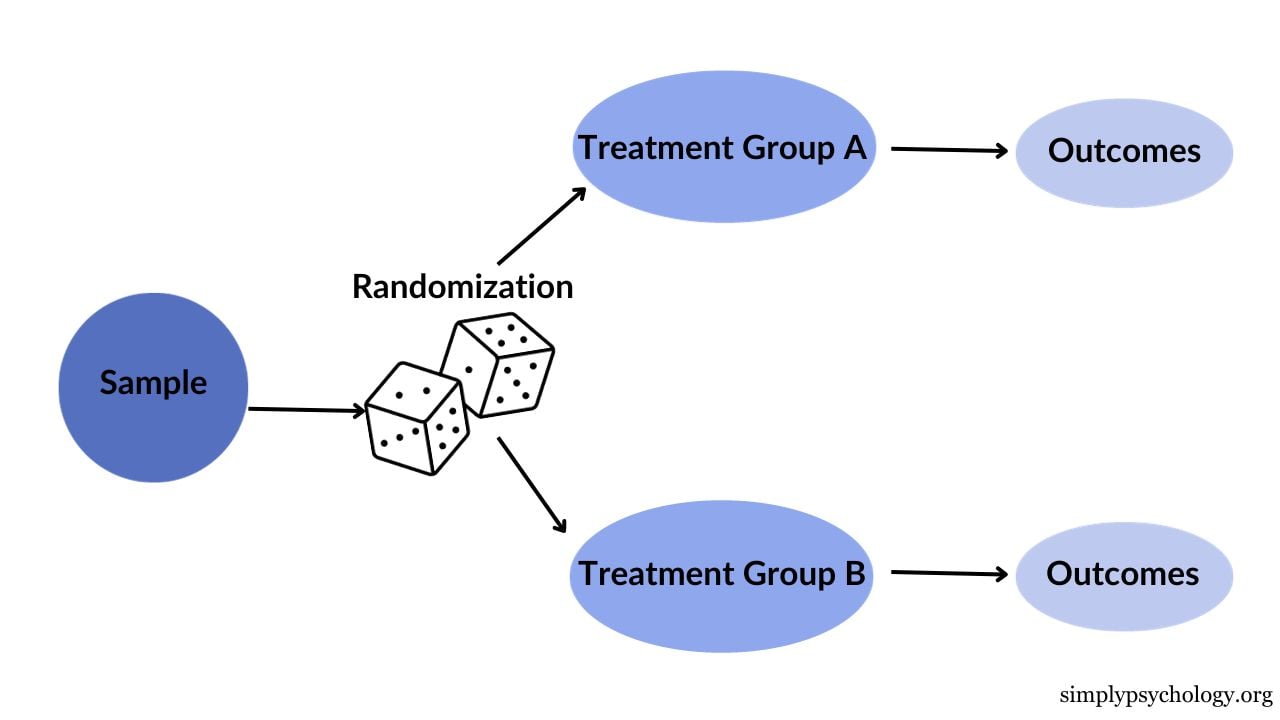
Random Selection
Randomly selecting participants helps prevent systematic differences between groups that could provide alternative explanations. It ensures any pre-existing factors are evenly distributed by chance, strengthening the ability to attribute results to the independent variable rather than confounds.
Blinding
Blinding (also called masking) refers to keeping trial participants, healthcare providers, and data collectors unaware of the assigned intervention so as not to be influenced by knowledge. This minimizes bias in instrumentation, drop-out rates (attrition), and participant bias.
Control Groups
Control groups are groups for whom an experimental condition is not applied. These show whether or not there is a clear difference in outcome related to the application of the independent variable.
The use of a control group in combination with randomized allocation constitutes a randomized control trial, which scholars consider to be a “gold standard” for psychological research (Kim & Shin, 2014).
Study protocol
Study protocols are pre-defined plans that detail all aspects of a study: experimental design, methodology, data collection and analysis procedures, and so on. This helps to ensure consistency throughout the study, reducing the effects of instrumentation and differential diffusion of information on internal validity (Kim & Shin, 2014).
Allocation concealment
In a research study comparing two treatments, participants must be randomly assigned so that neither the researchers nor participants know which treatment they will get ahead of time.
This process of hiding the upcoming assignment is called allocation concealment. It’s crucial because if researchers or participants know or influence which treatment someone will receive, it ruins the randomness.
For example, if a researcher believes one treatment is better, they may steer sicker participants toward it rather than assigning them fairly by chance.
Proper allocation concealment prevents this by keeping upcoming assignments hidden, ensuring unbiased random group assignments.
Internal Validity Example
A researcher hypothesizes that a new cognitive training program will improve memory and attention in elderly adults. To test this, the researcher randomly assigns older adults from the same retirement community to either the new training program (experimental group) or a general health education program (control group) for 8 weeks. The researcher double-blinds the experiment, so neither the participants nor test administrators know who is in each group. Participants are given memory and attention tests before and after the 8-week period. The researcher accounts for any potential confounding variables by collecting data on participants' baseline cognitive functioning, health status, age, education level, and gender. Strict protocols are used to deliver the cognitive and health education programs identically to minimize instructor biases. Statistical analyses check that any drop-outs do not differentially affect the groups. With these controls, the researcher can have strong internal validity to determine if observed improvements are due to the new cognitive training rather than other variables.
FAQs
What is the difference between internal and external validity?
Internal validity is a statement of causality and non-interference by extraneous factors, while external validity is a statement of an experiment’s generalizability to different situations or groups.
Why is internal validity more critical than external validity in a true experiment?
Internal validity concerns the robustness of an experiment in itself. An experiment with external but not internal validity cannot be used to conclude causality. Thus, it is generally unreliable for making any scientific inferences. On the contrary, an experiment that has only internal validity can be used, at least, to draw causal relationships in a narrow context.
References
American Psychological Association. Internal Validity. American Psychological Association Dictionary.
Blasco-Fontecilla, H., Delgado-Gomez, D., Legido-Gil, T., De Leon, J., Perez-Rodriguez, M. M., & Baca-Garcia, E. (2012). Can the Holmes-Rahe Social Readjustment Rating Scale (SRRS) be used as a suicide risk scale? An exploratory study. Archives of Suicide Research, 16(1), 13-28.
Brehm, J. W. (1966). A theory of psychological reactance.
Campbell, D. T. (1957). Factors relevant to the validity of experiments in social settings. Psychological bulletin, 54(4), 297.
Gerst, M. S., Grant, I., Yager, J., & Sweetwood, H. (1978). The reliability of the Social Readjustment Rating Scale: Moderate and long-term stability. Journal of psychosomatic research, 22(6), 519-523.
Holmes, T. H., & Rahe, R. H. (1967). The social readjustment rating scale. Journal of psychosomatic research, 11(2), 213-218.
Kevin J. Flannelly, Laura T. Flannelly & Katherine R. B. Jankowski (2018): Threats to the Internal Validity of Experimental and Quasi-Experimental Research in Healthcare, Journal of Health Care Chaplaincy, DOI: 10.1080/08854726.2017.1421019
Kim, J., & Shin, W. (2014). How to do random allocation (randomization). Clinics in orthopedic surgery, 6(1), 103-109.
Morse, G., & Graves, D. F. (2009). Internal Validity. The American Counseling Association Encyclopedia, 292-294.