On This Page:
Cluster sampling is typically used when the population and the desired sample size are particularly large.
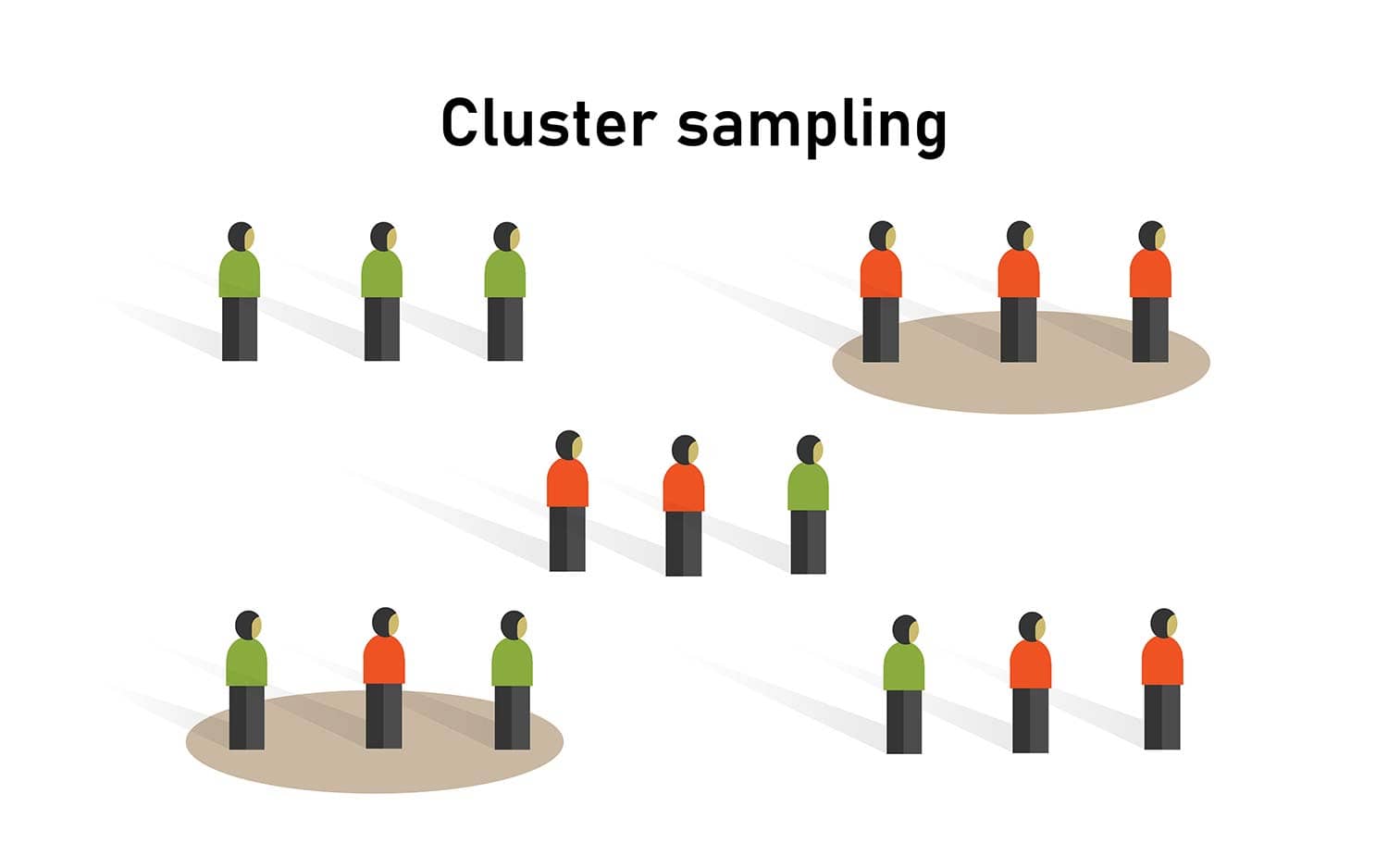
The purpose of cluster sampling is to reduce the total number of participants in a study if the original population is too large to study as a whole. These clusters serve as a small-scale representation of the total population, and taken together, the clusters should cover the characteristics of the entire population.
This sampling method reduces the cost and time of a study by increasing efficiency. Researchers sometimes will use pre-existing groups such as schools, cities, or households as their clusters.
Key Terms
- A sample is the participants you select from a target population (the group you are interested in) to make generalizations about. As an entire population tends to be too large to work with, a smaller group of participants must act as a representative sample.
- Representative means the extent to which a sample mirrors a researcher’s target population and reflects its characteristics (e.g., gender, ethnicity, socioeconomic level). In an attempt to select a representative sample and avoid sampling bias (the over-representation of one category of participant in the sample), psychologists utilize a variety of sampling methods.
- Generalisability means the extent to which their findings can be applied to the larger population of which their sample was a part.
Cluster Sampling Techniques
Single-stage cluster sampling
-
- A single-stage cluster is a type of cluster sampling where each unit of the chosen clusters is sampled. Researchers will first divide the total sample into a predetermined number of clusters based on how large they want each cluster to be.
- Then, they randomly select and sample from the clusters and collect data from each individual unit in the selected clusters.
Double-stage cluster sampling
-
- In two-stage cluster sampling, researchers will only collect data from a random subsample of individual units within each of the selected clusters to use as the sample.
- This technique is less precise than single-stage sampling and should only be used when it is too challenging or expensive to test the entire cluster.
Multi-stage cluster sampling
-
- This type of cluster sampling involves the same process as double-stage sampling, except with a few extra steps.
- In multi-stage sampling, researchers will continue to randomly sample elements from within the clusters until they reach a manageable sample size.
Applications
Cluster sampling is used when the target population is too large or spread out, and studying each subject would be costly, time-consuming, and improbable.
Cluster sampling allows researchers to create smaller, more manageable subsections of the population with similar characteristics. Cluster sampling is particularly useful in areas of geographical sampling when the populations are widely dispersed.
Researchers will form clusters based on a geographical area by grouping individuals within a community, neighborhood, or local area into a single cluster.
Cluster sampling is also used in market research when researchers cannot collect information about the population as a whole. Lastly, cluster sampling can be used to estimate high mortality rates, such as from wars, famines, or natural disasters.
How to Cluster Sample?
- First, choose the target population that you wish to study and determine your desired sample size.
- Then, divide your sample into clusters. When forming the clusters, make sure each cluster’s population is diverse, has a similar distribution of characteristics to the distribution of the population as a whole, and has the same number of members. The goal is to form clusters that are representative of the total population as a whole.
- Next, select clusters by a random selection process. It is important to randomly select from the clusters to preserve your results’ validity. The number of clusters selected is based on how large the sample size is.
- In single-stage sampling, collect data from each individual unit of the clusters you selected in Step 3.
- In the case of double-stage or multi-stage sampling, you randomly select individual units from within the selected clusters to use as your sample. You will then collect your data from each of these individual units. Double-stage and multi-stage clustering tend to be easier than single-stage because you will work with a much smaller sample.
Advantages
Time and cost-efficient
Cluster sampling is cheaper and quicker than other sampling methods. For example, it reduces travel expenses for wide geographical populations.
High external validity
If your population is clustered properly to represent every possible characteristic of the entire population, your clusters will accurately reflect the entire population.
Practicality and ease
This type of sampling process enables researchers to study large populations that would otherwise be too challenging or complicated to analyze otherwise.
Limitations
High sampling error
When the clusters do not mirror the population’s characteristics or serve as a mini-representation of the population as a whole, there will be less statistical certainty and accuracy. This error is even greater when you use more stages of clustering.
Complexity
Planning study designs for cluster sampling usually requires more attention because researchers need to determine how to divide up a larger population efficiently and properly.
Example Situations
- Assess immunization coverage (Henderson & Sundaresan, 1982).
- Estimate density of waterfowl wintering (Smith, Conroy, & Brakhage, 1995).
- Conduct a rapid assessment of health in communities affected by natural disasters (Malilay, Flanders, & Brogan, 1996).
- Determine forest inventories (Roesch, 1993).
- Assess the prevalence of irritable bowel syndrome in South China and its impact on health-related quality of life (Xiong, 2004).
- Estimate the size of hidden and hard to access populations (Medina & Thompson, 2004).
Cluster Sampling vs. Stratified Sampling
Stratified sampling is a method where researchers divide a population into smaller subpopulations known as a stratum. Stratums are formed based on shared, unique characteristics of the members, such as age, income, race, or education level.
Then, members of the strata are randomly selected to form a sample.
Researchers using stratified sampling divide the population into groups based on age, religion, ethnicity, or income level and randomly choose from these strata to form a sample.
Alternatively, researchers using cluster sampling will use naturally divided groups to separate the population (i.e., city blocks or school districts) and then randomly select elements from these clusters to be a part of the sample.
References
Felix-Medina, M. H., & Thompson, S. K. (2004). Combining link-tracing sampling and cluster sampling to estimate the size of hidden populations. JOURNAL OF OFFICIAL STATISTICS-STOCKHOLM-, 20 (1), 19-38.
Henderson, R. H., & Sundaresan, T. (1982). Cluster sampling to assess immunization coverage: a review of experience with a simplified sampling method. Bulletin of the World Health Organization, 60 (2), 253–260.
Malilay, J., Flanders, W. D., & Brogan, D. (1996). A modified cluster-sampling method for post-disaster rapid assessment of needs. Bulletin of the World Health Organization, 74 (4), 399–405.
Roesch, F. A. (1993). Adaptive cluster sampling for forest inventories. Forest Science, 39 (4), 655-669.
Smith, D. R., Conroy, M. J., & Brakhage, D. H. (1995). Efficiency of Adaptive Cluster Sampling for Estimating Density of Wintering Waterfowl. Biometrics, 51 (2), 777–788. https://doi.org/10.2307/2532964
Steven K. Thompson (1990) Adaptive Cluster Sampling, Journal of the American Statistical Association, 85:412,1050-1059, DOI: 10.1080/01621459.1990.10474975
Xiong, L. S., Chen, M. H., Chen, H. X., Xu, A. G., Wang, W. A., & Hu, P. J. (2004). A population‐based epidemiologic study of irritable bowel syndrome in South China: stratified randomized study by cluster sampling. Alimentary pharmacology & therapeutics, 19 (11), 1217-1224.
Further Information
Sedgwick, P. (2014). Cluster sampling. Bmj, 348.
Marketing researchers often use city blocks as clusters in cluster sampling. Using this fact, explain how a market researcher might use multistage cluster sampling to select a sample of consumers from all cities having a population of more than 10,000 .
In multistage cluster sampling, the process begins by dividing the larger population into clusters, then randomly selecting and subdividing them for analysis.
For market researchers studying consumers across cities with a population of more than 10,000, the first stage could be selecting a random sample of such cities. This forms the first cluster.
The second stage might randomly select several city blocks within these chosen cities – forming the second cluster.
Finally, they could randomly select households or individuals from each selected city block for their study. This way, the sample becomes more manageable while still reflecting the characteristics of the larger population across different cities.
The idea is to progressively narrow the sample to maintain representativeness and allow for manageable data collection.
When is cluster sampling appropriate?
Cluster sampling is appropriate when:
1. The population is widespread geographically, and conducting simple random sampling is costly or impractical. Clusters can be geographically based to minimize travel costs.
2. Data collection involves face-to-face interviews or on-site inspections.
3. A list of individuals in the population is unavailable, but it’s possible to identify clusters representing the population.
4. The population is naturally divided into groups (clusters), and these clusters are internally heterogeneous, i.e., they reflect the diversity of the overall population.
It provides a balance between statistical accuracy and cost-effectiveness in such cases.
What is a cluster sample?
A cluster sample is a sampling method where the researcher divides the entire population into separate groups, or clusters. Then, a random sample of these clusters is selected. All observations within the chosen clusters are included in the sample. This method is typically used when the population is large, widely dispersed, and inaccessible. The clusters should ideally mirror the characteristics of the population as a whole.