On This Page:
The availability heuristic is a cognitive bias in which you make a decision based on an example, information, or recent experience that is that readily available to you, even though it may not be the best example to inform your decision (Tversky & Kahneman, 1973).
In other words, information that is more easily brought to mind (i.e., more available) is assumed to reflect more frequent and/or more probable events.
While the information that is more difficult to bring to mind (i.e., less available) is assumed to reflect less frequent and/or less probable events.
Consider, for example, a person trying to estimate the relative probability of owning a dog versus owning a ferret as a household pet. In all likelihood, it is easier to think of an example of a dog-owning household than it is to think of an example of a ferret-owning household.
Therefore, a person in this situation may (correctly) reason that the former four-legged is considerably more common as a household pet.
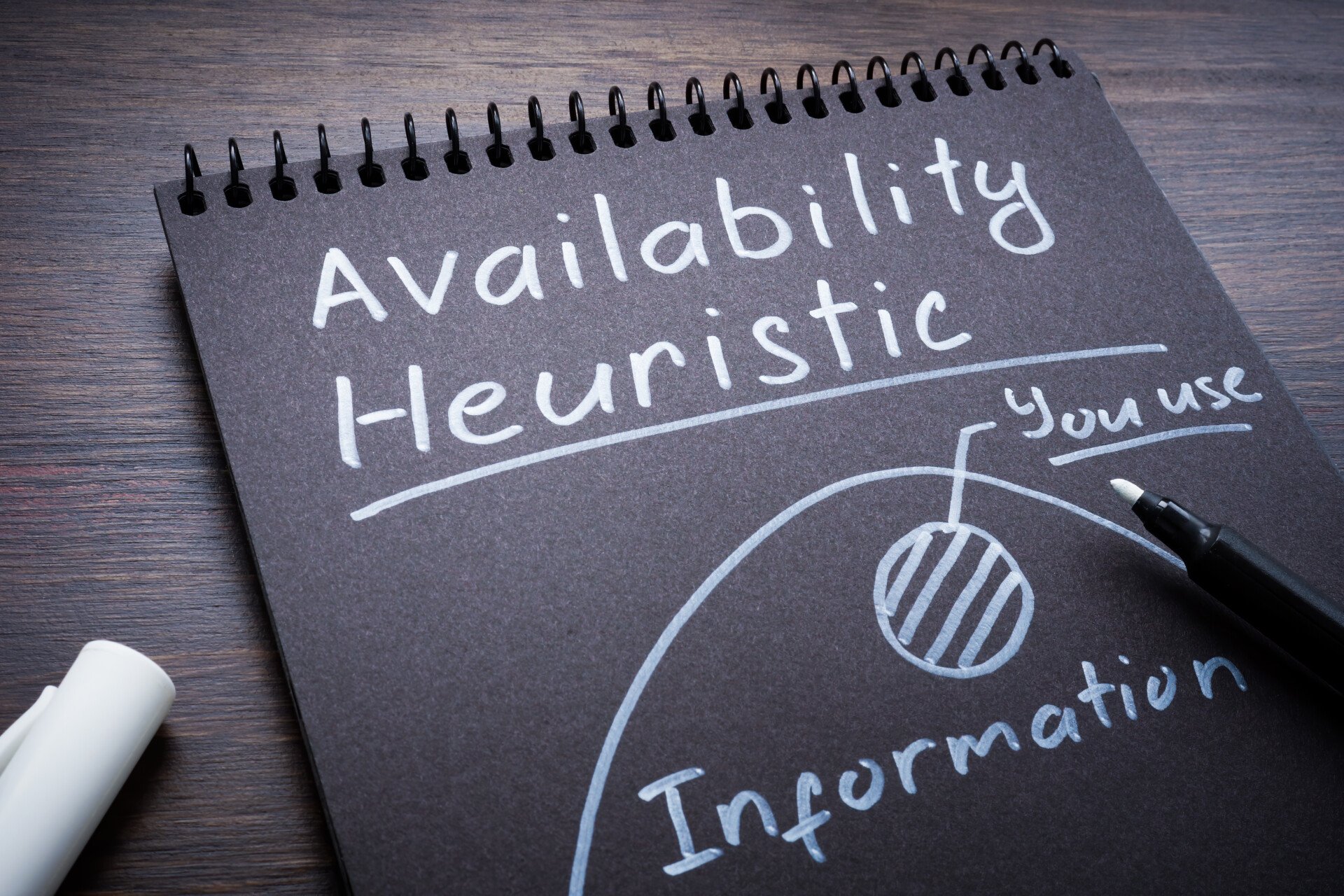
It is often the case that more frequent events are indeed more easily recalled than less frequent events, and so this mental manipulation regularly leads to rapid and accurate judgments in a range of real-world scenarios (Markman & Medin, 2002).
However, the availability bias is also prone to predictable errors in certain situations and thus is not always a reliable shortcut for decision-making.
Historical Background
- The availability bias belongs to a larger framework of heuristics and cognitive biases within behavioral economics or the interdisciplinary study of human behavior and decision-making (American Psychological Association).
- A holistic understanding of the availability bias requires acknowledging the theories and models that have defined research in this discipline since the turn of the twentieth century.
- In the early 1900s, behavioral economic research assumed that humans were entirely rational actors in decision-making, as defined by the purely economic model of rationality. This model proposed that when making decisions, humans were able to accurately assess all available options and information in order to make optimal judgments. Errors in judgment were thus both unexpected and unexplainable (Gilovich et al., 2002).
- A contribution by Herbert Simon in the 1950s helped to make sense of the seemingly unsystematic errors of supposedly rational decision-makers. Simon introduced the idea of bounded rationality, which proposed that humans attempt to make the best decisions possible within the intrinsic constraints of their own processing power.
- In other words, it is not always possible to accurately consider all relevant information when making a decision; in these cases, humans work with the most available and relevant information (Simon, 1957, as cited in Gilovich et al., 2002).
- This concept of bounded rationality laid the foundation for discussing heuristics and biases, or the mental shortcuts of decision-making.
- Psychologists Daniel Kahneman and Amos Tversky produced the most notable early contributions to this field. Kahneman and Tversky (1974) recognized, categorized, and empirically analyzed a set of heuristics used in decision-making scenarios in which not all information was accessible, otherwise known as scenarios of the judgment under uncertainty.
- Their original research on judgment under uncertainty focused on the availability, representativeness, and anchoring/adjustment biases. Much of Kahneman and Tversky’s original research on these biases is still widely cited by behavioral economists today.
How the Availability Heuristic Works
The human brain is eager to use whatever information it can to make good decisions. However, obtaining all relevant information in decision-making scenarios is not always easy, nor even possible.
And even in situations in which all relevant information is available, analyzing all potential options and outcomes is computationally expensive.
Therefore, the brain takes frequent and predictable shortcuts. The availability bias – in which the prevalence and likelihood of an event are estimated by the ease with which relevant examples can be recalled – is one such mental shortcut.
Thus, this heuristic allows people to make fast and accurate estimations in many real-world scenarios. However, there are certain predictable moments in which less frequent events are easier to recall than more frequent – such as in the examples listed below – and so the availability bias errs (Markman & Medin, 2002).
Markman and Medin (2002) help to explain this phenomenon by providing an analogy of another useful system of shortcuts that occasionally leads to faulty judgment: the human visual system.
Undeniably, the human perceptual system is incredibly refined and extremely useful. However, because of the shortcuts this system often takes to provide the brain with understandable visual input, it is still prone to error in the case of optical illusions.
Examples
Here are a few scenarios where this could play out in your day-to-day life.
Winning the Lottery
Hundreds of millions of people participate in lotteries every year, and yet by definition, very few are successful. So why do people continue to play?
The availability bias can help to explain why people have an unfortunate tendency to severely misjudge their personal probability of winning the lottery.
The probability of winning the Powerball jackpot lottery is approximately 1 in 300 million (Victor, 2016).
However, given that it is easier to bring to mind images of lottery winners (and their winnings) than lottery losers (and their lack thereof), it is subconsciously believed that winning the lottery is a far more likely occurrence than it actually is (Griffiths & Wood, 2001; Kahneman, 2011).
Safety
It is common for people to overestimate the risk of certain events (such as plane crashes, shark attacks, and terrorist attacks) while underestimating the risk of others (such as car crashes and cancer).
For example, many people are warier of traveling by plane than by car and may even opt to drive rather than fly when possible out of concern for personal safety.
In reality, it has been calculated that driving the distance of an average flight path is 65 times riskier than flying itself (Sivak & Flannigan, 2003).
Fear of shark attacks is another common public safety concern, despite actual attacks being incredibly rare. The International Shark Attack File estimates that the risk of death due to a shark attack is over 1 in 3.7 million (to put this into perspective, being fatally struck by lightning, another extraordinarily rare occurrence, is about 47 times more likely) (“Risk of death,” 2018).
The overestimated risk of events such as these is often related to their sensationalized media coverage, which causes associated examples and images to be readily brought to mind.
On the other hand, more common occurrences such as car crashes often do not receive the same media attention and thus are less readily mentally available (Kahneman, 2011).
The availability bias, as it applies to safety concerns, can also help to explain the spending patterns of the United States federal budget.
Despite cancer being a far greater risk to American lives than events such as terrorist attacks, the annual funding directed toward cancer research only equates to a tiny fraction of the United States defense and military budget (“Federal spending,” n.d.; “Risk of death,” 2018).
Insurance Rates
After natural disasters (i.e., floods), it has been observed that related insurance rates (i.e., the rate at which consumers purchase flood insurance) spike in affected communities.
It can be reasoned that the experience of disaster causes community members to reevaluate their perceived risk of danger and to protect themselves accordingly.
However, it has likewise been observed that in the years following these disasters, insurance rates steadily declined back to baseline levels, despite disaster risk in the community remaining the same throughout the entire time period (Gallagher, 2014).
In these cases, it is not only the risk of the disaster itself but the ease with which the experience of disaster is brought to mind that influences a community member’s decision to purchase the relevant protective insurance.
In other words, since it is easier to recall the experience of a disaster that occurred recently, community members are likely to overestimate the risk of a repeated event in the years immediately following the disaster.
On the other hand, since it is more difficult to recall the experience of a disaster that occurred in the distant past, community members are likely to underestimate the risk of a repeated event several years after the disaster.
This pattern of overestimation and underestimation of risk is the result of the availability bias and can explain the spiking and declining insurance rate patterns observed in disaster-struck communities (Gallagher, 2014; Kahneman, 2011).
Self-Evaluation
Schwarz et al. (1991) sought to distinguish whether the availability bias operated on the content of recall (i.e., the number of instances recalled) or ease of recall (i.e., how easy or hard it is to recall those instances).
To test this, they designed a clever study in which participants were tasked with listing either 6 or 12 examples of assertive behaviors and then asked to rate their own assertiveness on a scale from one to ten.
When the data were analyzed, it was found that participants who were tasked with listing six examples of assertive behaviors rated themselves as significantly more assertive than those tasked with listing 12 assertive behaviors. Why?
When participants only had to list six examples of assertive behaviors, the fact that it was relatively easy to do so led participants to believe that they must be assertive if it was so easy to accomplish this task.
On the other hand, when participants had to list 12 examples of assertive behaviors, the fact that it was relatively difficult to do so led participants to believe that they must not be that assertive if it was so difficult to accomplish this task.
This study demonstrated that the availability bias operates not on the content of recall (i.e., number of instances recalled) but on ease of recall (i.e., how easy or hard it is to recall those instances).
Participants did not measure their own assertiveness with respect to the total number of instances recalled but rather with respect to the ease (or lack thereof) with which these instances came to mind (Schwarz et al., 1991).
If the opposite were true – that is, if the availability bias operated on the content of recall as opposed to the ease of recall – then it would have been found that participants tasked with listing more examples of assertive behaviors (i.e., 12 examples) would likewise rate themselves as more assertive than those tasked with listing fewer examples of assertive behavior (i.e., six examples). This was not the case.
Course Evaluation
As a follow-up study to the research by Schwartz et al. on self-perceptions of assertiveness, Fox (2006) tested the availability bias on graduate students in a business course at Duke University.
On a mid-course evaluation survey, Fox assigned half the class to list two potential improvements to the course and the other half to list ten. Both halves then had to provide an overall class rating.
As expected, students tasked with listing two-course improvements (a relatively easy task) rated the course more negatively than students tasked with listing ten-course improvements (a relatively difficult task).
In other words, when students only had to list two suggestions for course improvements, the fact that it was relatively easy to do so led participants to believe that the course must need improvement if it was so easy to accomplish this task (and thus provided a lower course rating).
On the other hand, when students had to list ten suggestions for course improvements, the fact that it was relatively difficult to do so led participants to believe that the course must not need much improvement if it was so difficult to accomplish this test (and thus provided a higher course rating).
This once again demonstrates that the availability bias operates with respect to ease of recall, not the content of recall (Fox, 2006).
Word Frequency
In their earliest research paper on the availability bias, Kahneman and Tversky asked readers to consider whether there exist more words that begin with the letter k or words that have the letter k as their third letter. (Try to answer this yourself before reading on!)
A reasonable attempt to answer this question may involve bringing to mind examples in each category. Since it is considerably easier to think of words that begin with k than to think of words that have k as their third letter, it is commonly assumed that there are many more words in the former category (words that begin with the letter k).
However, the opposite is true, and in fact, there are approximately twice as many words that have k as their third letter. This is a situation in which the use of the availability bias results in a predictable error.
Given the way that humans categorize words and letters, it is far easier to search for words by their first letter than by their third. Therefore, words beginning with the letter k are more easily brought to mind and are likewise assumed to be more frequent occurrences (Kahneman & Tversky, 1974; Tversky & Kahneman, 1973).
Implications
Though the availability bias often leads to accurate judgments in a range of real-world scenarios, it is still prone to error in certain predictable situations.
In these situations, the use of availability bias can lead to faulty judgment. These errors in judgment can have a significant and rapid impact on human behavior – sometimes with negative consequences.
Politics
Politicians can (and often do) use the availability bias for their own political gain. By overemphasizing certain issues, threats, or even the negative qualities of an opposing candidate, they can make people believe that these things are more frequent and relevant than they actually are.
Marketing
Marketing companies can use availability bias to increase their profits. By overemphasizing the downsides of not buying a particular product, they can convince customers that their need for the said product is greater than it actually is.
Evaluation of Self
As seen in the assertiveness study by Schwarz et al. (1991), the availability bias can impact students’ evaluations of their own assertiveness (see ‘Examples – Self-Evaluation’). Though this particular study focused only on the character trait of assertiveness, it can be reasoned that this effect would also be present with other character trait evaluations.
Evaluation of Others
Memorable interactions with others in which a certain characteristic is prominently displayed (e.g., when a person is particularly rude, or particularly clumsy) can cause people to imagine that these characteristics are more common in the other person than they actually are.
Education
As seen in the course evaluation study by Fox (2006), the availability bias can impact students’ evaluations of their own education (see ‘Examples – Course Evaluation’).
Given that students’ use of the availability bias had an immediate and significant impact on their overall course evaluation, this particular study also provides a demonstration of how quickly and efficiently the availability bias can work.
Social Media
The social media trend for posts to be more positive than negative (i.e., more likely to be of happy moments than of sad moments) may cause viewers to overestimate the happiness of others and to underestimate their own in comparison.
Overcoming the Availability Bias
Bear in mind that in many cases, the availability bias leads to correct frequency and probability estimations in real-world scenarios, and so it is neither recommended (nor likely even possible) to overcome the use of the bias entirely.
However, the potentially negative effects of the availability bias can be mitigated by remembering to consider all relevant data when making judgments under uncertainty, not just that which comes readily to mind.
Indeed, it is possible to become a more thoughtful decision-maker by simply recognizing the predictable situations in which the availability bias may err and lead to faulty judgment. And with that in mind, you are already one step ahead by reading this article!
Critical Evaluation
The availability bias faced critical evaluation by Schwarz et al. (1991) for being ambiguous in terms of its specific underlying process.
Specifically, Schwarz et al. sought to distinguish whether the frequency and probability judgments were the result of the content of recall (i.e., the number of instances recalled) or ease of recall (i.e., how easy or hard it is to recall those instances).
This theoretical question resulted in their famous study on self-perceptions of assertiveness, in which it was found that participants who were tasked with listing six examples of assertive behaviors rated themselves as significantly more assertive than those tasked with listing 12 assertive behaviors (see ‘Examples – Self-Evaluation’).
This study thus demonstrated that the availability bias operated on ease of recall, not the content of recall (Schwarz et al., 1991).
Related Cognitive Biases
The availability bias is one of several cognitive biases, or mental shortcuts, used in judgment-making scenarios. Two other common biases are the representativeness bias and the anchoring/adjustment bias.
These three biases together served as the primary focus of Kahneman and Tversky’s seminal work on judgment under uncertainty, and each remains central to the discussion of decision-making today (Kahneman & Tversky, 1974).
Each bias has a distinct definition and a unique set of common examples of its usage and error. However, it is noteworthy that there are moments in which two or more biases may be used in conjunction.
In other words, decisions are not necessarily influenced by only one bias at a time, and may instead be the result of the influence of multiple biases.
The human-decision making process is multifaceted in nature and can also be influenced by factors such as individual differences and emotional response (Payne et al., 1993; Slovic et al., 2007).
As such, an attempt at a holistic discussion of decision-making would necessitate a much longer article.
However, biases such as the availability bias, the representativeness bias, and the anchoring/adjustment bias nevertheless provide useful and interesting insight into the processes of the human mind during judgment-making scenarios.
Representativeness Bias
The representativeness bias (also known as the representativeness heuristic) is a common cognitive shortcut used for making judgments of probability, in which the likelihood of an occurrence is estimated by the extent to which it resembles (i.e., is representative of) an exemplary occurrence (Kahneman & Tversky, 1974).
In other words, the more similar an example occurrence A is to our preconceived idea of a model occurrence B, the more likely it is considered to be. On the other hand, the more dissimilar an example occurrence A is from our preconceived idea of a model occurrence B, the less likely it is considered to be.
A common example of representativeness bias concerns the concept of randomness. Consider a coin toss sequence in which H represents a coin landing on heads and T represents a coin landing on tails. The sequence H-T-T-H-T-H is considered more likely than the sequence H-H-H-T-T-T because the former sequence more closely resembles our preconceived idea of randomness.
In reality, given that the probability of a coin landing on either side is always 50% (0.5), the likelihood of the sequence is exactly the same (0.5 x 0.5 x 0.5 x 0.5 x 0.5 x 0.5 = 0.56 = 0.015625, or about 1.5%) (Kahneman & Tversky, 1974).
Anchoring/Adjustment Bias
The anchoring/adjustment bias (also known as the anchoring/adjustment heuristic) is a common cognitive shortcut used for making evaluations and estimations, in which assessments are made by adjusting from an initial reference point (or anchor).
This adjustment is often insufficient, and occurs even in situations in which the reference point is entirely unrelated to the estimation (Kahneman & Tversky, 1974).
In other words, people have a tendency to overvalue initial information, regardless of relevance, when making evaluations and estimations. For example, consider a retail item that costs $100.
This price is more likely to be seen as reasonable if the item is currently on sale from an original price of $200 than if the price recently increased from $50 to $100 (or even if the price remained consistent at $100).
Though the final price is identical in each of these scenarios ($100), the evaluation of its reasonableness varies considerably based on the initial price, which serves as a mental anchor.
Notably, this phenomenon can be observed even in scenarios where the anchor is irrelevant to the evaluation. Kahneman and Tversky (1974) famously demonstrated this effect by asking subjects whether the percentage of African countries in the United Nations was higher or lower than 65% (for one group) or 10% (for another group), and then asking them to provide an exact estimation.
Subjects who were anchored to the number 65 provided significantly higher estimates for the percentage of African countries in the United Nations than subjects who were anchored to the number 10 (with median estimates of 45% and 25%, respectively).
References
American Psychological Association. (n.d.). APA Dictionary of Psychology. https://dictionary.apa.org/behavioral-economics
Federal spending: Where does the money go. (n.d.). National Priorities Project. https://www.nationalpriorities.org/budget-basics/federal-budget-101/spending/
Fox, C. R. (2006). The availability heuristic in the classroom: How soliciting more criticism canboost your course ratings. Judgment and Decision Making, 1(1), 86-90.
Gallagher, J. (2014). Learning about an infrequent event: evidence from flood insurance take-upin the United States. American Economic Journal: Applied Economics, 206-233.
Gilovich, T., Griffin, D., & Kahneman, D. (Eds.). (2002). Heuristics and biases: The psychology of intuitive judgment. Cambridge university press.
Griffiths, M., & Wood, R. (2001). The psychology of lottery gambling. International gambling studies, 1(1), 27-45.
Kahneman, D. (2011). Thinking, fast and slow. Macmillan.
Kahneman, D., & Tversky, A. (1974). Judgment under uncertainty: Heuristics and biases. Science, 185, 1124–1131.
Markman, A. B., & Medin, D. L. (2002). Decision making.
Payne, J. W., Bettman, J. R., & Johnson, E. J. (1993). The adaptive decision maker. Cambridge university press.
Risk of death. Florida Museum. (2018). https://www.floridamuseum.ufl.edu/shark-attacks/odds/compare-risk/death/
Schwarz, N., Bless, H., Strack, F., Klumpp, G., Rittenauer-Schatka, H., & Simmons, A. (1991). Ease of retrieval as information: Another look at the availability heuristic. Journal of Personality and Social Psychology, 61, 195–202.
Sivak, M., & Flannagan, M. J. (2003). Macroscope: flying and driving after the september 11 attacks. American Scientist, 91(1), 6-8.
Slovic, P., Finucane, M. L., Peters, E., & MacGregor, D. G. (2007). The affect heuristic. European journal of operational research, 177(3), 1333-1352
Tversky, A., &l Kahneman, D. (1973). Availability: A heuristic for judging frequency and probability. Cognitive psychology, 5(2), 207-232.
Victor, D. (2016). You will not win the powerball jackpot. The New York Times. https://www.nytimes.com/2016/01/13/us/powerball-odds.htm